Rapid Deep Learning-based Flood Losses/Risk Prediction Tool (RAPFLO)
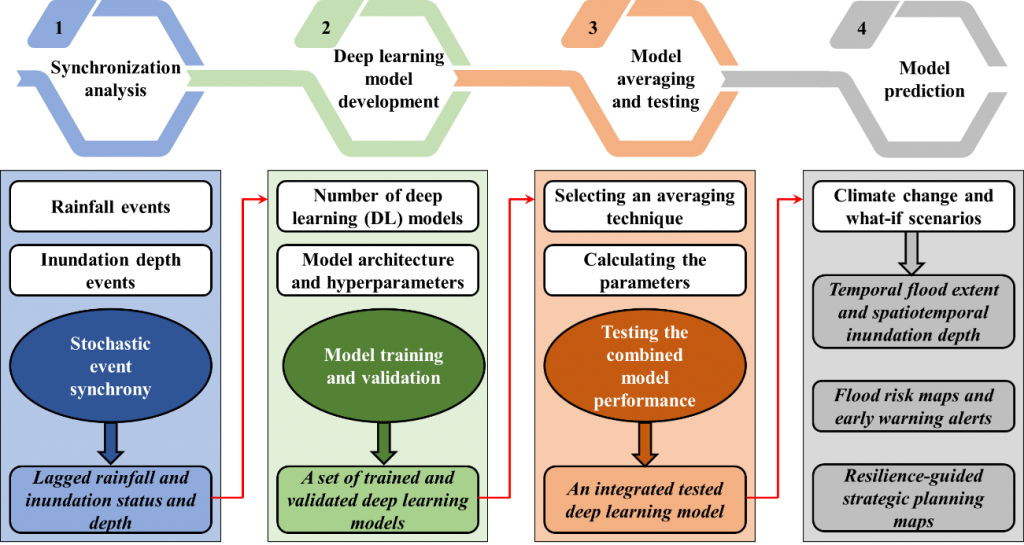
Tech ID
23-078
Inventors
Wael El-Dakhakhni
Patent Status
US provisional filed
Stage of Research
Tool was used to recreate fluvial flood risk in the city of Calgary
Contact
Lokesh Mohan
Business Development Officer
Abstract
Floods, among the costliest and most destructive weather-related extremes, result in significant casualties, economic losses, and lasting health issues. The complex interplay of meteorological, physical, and anthropogenic factors determines flood risk. System vulnerability, considering social, economic, and physical aspects, plays a crucial role in flood risk assessment. Weighting factors based on expert knowledge or data-driven methods help determine their relative importance. Flood hazard, evaluated through physics-based or data-driven approaches, requires complex hydrologic modeling and hydraulic simulation, often posing computational challenges. Data-driven models correlate location-specific flooding frequency with hydrologic and topographic factors using statistical or machine learning techniques. Deep learning models offer efficient alternatives but require climate model integration for accurate flood risk prediction under climate change. However, in order to accurately estimate the fluvial flood risk under climate change, a continuous real-time simulation is required which typically requires an extensive amount of data and processing time.
Researchers at the McMaster have developed a rapid accurate prediction of flood risk (RAPFLO) tool that uses hierarchical deep neural networks to estimate fluvial flood risk likelihood and level. RAPFLO’s generic nature allows it to be applied to different areas with appropriate data availability. The underlying methodology of the RAPFLO aims at directly estimating the fluvial flood risk level and likelihood based on implicitly simulating the complex interactions between the factors associated with the flood hazard and the built system vulnerability. The tool was utilized to recreate the fluvial flood risk in the city of Calgary, Canada, spanning from 2010 to 2020. It effectively replicated the risk level with an overall accuracy rate of 80% and the likelihood with a coefficient of determination of 0.96. This developed tool offers a computationally efficient, precise, and swift decision support system. It empowers city managers and infrastructure operators to devise effective climate resilience strategies while considering various climate projections and potential future scenarios.
Applications
- Flood risk prediction/warning
- Climate Change
- City Planning
- Insurance
Benefits
- Accurate prediction
- Computationally efficient
- Significant reduction in time spent and resources used
- Effective alternative to uncertain physics-based models and tedious manipulations
References
- Ghaith, M.; Yosri, A.; El-Dakhakhni, W. Synchronization-Enhanced Deep Learning Early Flood Risk Predictions: The Core of Data-Driven City Digital Twins for Climate Resilience Planning. Water 2022, 14, 3619. https://doi.org/10.3390/w14223619